AI's potential to revolutionize products is immense—but it comes with both risks and opportunities. At AnswerLab, we bel...
Informing Responsible AI Through UX Research
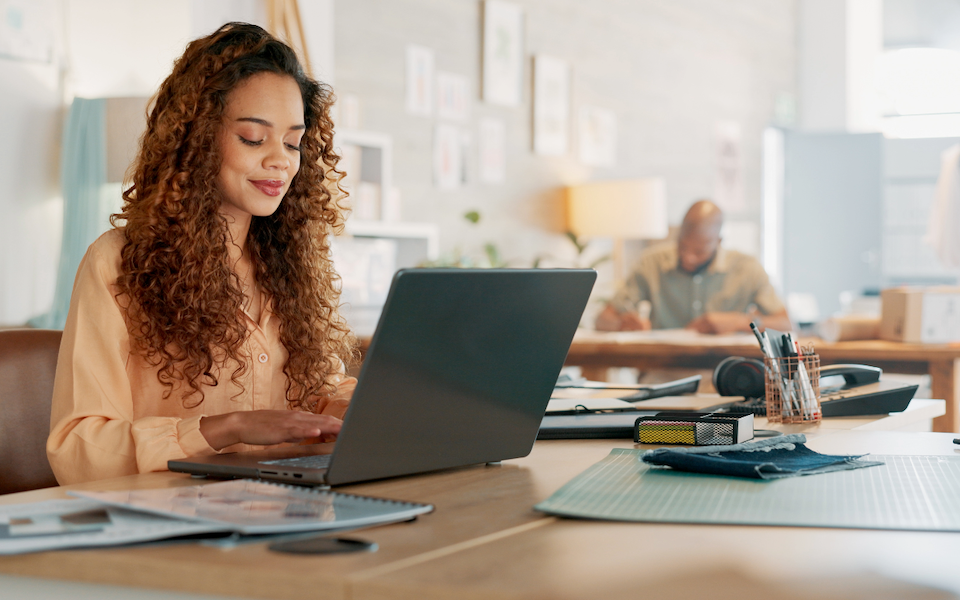
Posted by Max Symuleski on Sep 1, 2023
The author published an updated article on this topic in 2024 - check it out here: Building AI Products Responsibly: Why Product Research Matters.
AI is powerful—with potential not only for good but for malicious and unintended harm. Companies need to take steps to implement responsible AI practices. This article will share our recommendations for UX research aimed at building responsible AI practices.
Why “Responsible” AI?
With all the excitement around AI, it can be easy to get swept away in the capabilities and innovation it could bring to your product experiences. But utilizing and implementing AI comes with risks. As we develop new AI-powered experiences, we must be aware of the profound impacts it can have on individuals and society and take steps to ensure we're building our future intentionally.
How can AI cause harm?
AI training data can perpetuate and amplify bias, discrimination, and inequality. Models trained on that data can reinforce and prolong prejudiced patterns. For example, the levels to which financial services companies use AI in their application and approval systems can reinforce stereotypes and economic inequalities.
There are also potential threats to privacy and security due to the mass amounts of data being collected and surveilled through AI processes. There’s always a possibility that this data could be stolen or misused depending on the level of your security protocols.
The bottom line here is AI is powerful—and there’s potential for both malicious or mere unintended use cases that could harm users.
We need responsible AI management.
As AI becomes more ubiquitous in the products and experiences we’re using day-to-day, organizations must actively manage these risks through responsible AI protocols and techniques. Developing processes to assess and regularly audit AI systems is a critical step towards fostering a sense of trust with users, complying with both existing and future regulations, and following ethical principles and practices to avoid harm.
Risks and harms are inherent in AI technology, but can also arise through specific use-cases, unintended uses, and context-specific entanglements with ramifications well beyond the realm of AI or computation. AI is “socio-technical”— it is always entangled with human institutions, activities, and interactions through its use in specific contexts.
How are companies tackling Responsible AI today?
There are a number of companies building principles to actively manage risks and harms that could arise from AI, from Microsoft to Google to Meta. While there are many similarities across these principles, these examples illustrate how product and industry-specific considerations affect how companies prioritize and talk about AI principles.
Meta’s top principle focuses on privacy and security due to the vast amount of data they collect from their users. LinkedIn’s top principles centers on economic advancement. Wired Magazine focuses on telling users how they will utilize AI in the context of journalism, sharing that they won’t publish stories with text generated or edited by AI tools.
While all of these brands state their principles differently, we see 5 top principles across these policies:
- Fairness to prevent and mitigate discrimination and bias.
- Privacy and Security to protect user data from misuse and external threats.
- Transparency to promote clarity and trust with users by giving information about how and when AI is in use and how it makes decisions and produces outputs.
- Accountability and Governance to prioritize the responsibility and role of organizations in deploying AI solutions. This includes compliance with regulations both current and future.
- Safety and Reliability to ensure AI is being used in a safe and reliable way through specific checkpoints and standards.
UX research is a critical step in your AI development journey.
Now that we’ve covered an overview of why we need responsible AI, let’s dive into some solutions as you start to tackle the question yourself. UX research is a critical step in your AI development journey. Speaking with people from diverse backgrounds helps you understand end-user needs to inform product development, building better products that lead to successful adoption. It also plays a critical role in understanding user concerns, assessing risks and harms, and preparing for new regulations around AI technology.
Research with AI experts
One way to get feedback on your responsible AI practices is by conducting research with experts in artificial intelligence. These participants might include experts from AI think tanks, computer scientists, industry experts, or professors in computer science or machine learning departments at colleges and universities. Expert reviews of your early concepts and drafts of AI principles, as well as how you’re communicating with users through help text and online resources, can help you identify where you might be lacking in transparency, missing a critical piece of the puzzle, or not explaining a concept well enough. Academic experts in AI ethics can help you evaluate how you’re communicating concepts with users, where you should be surfacing this kind of information, if your help text adequately explains how machine learning works, and more. They can also offer suggestions on the kinds of controls and agency users should have in AI-powered experiences.
Research with company employees on internal processes
Depending on the level of your own internal AI review processes, you might also consider doing some internal research with your own employees. This is especially applicable in situations where you are training machine learning models or developing AI-enabled products. Often, the people who are reviewing safety and privacy considerations around AI are not dedicated machine learning researchers. They might be product managers, software engineers, or lawyers, and you may need to spend extra time considering how to set them up for success, which in turn reduces harm for your users. It can be highly valuable to understand how you can better support your own internal employees who might be jumping head first into AI without a deep background in it. Questions might look like, “How can we make this process better for you?,” “What resources do you need to help identify issues and harms?,” or “How can we help you feel more confident in your decisions?”
Research with real users
Finally, and often, most obviously, talk with the people using your product and interacting with your AI tools! There is a trove of research to be done on users' perceptions of AI, how they understand its role in your product, and what their needs and concerns are. This is a significant part of building a successful AI system.
We’ve conducted a number of studies with our clients’ users on how they think about AI harms and even categorizing them to better understand users’ top concerns. You can use a range of methodologies here from classic one-on-one interviews to card sorts to diary studies. Diving deep with your users can help inform and prioritize risk mitigation efforts to address top concerns and questions.
Researching transparency
We recommend conducting research on the level of transparency and communication users want as a way to understand where your users are in their AI journey. These conversations can help you get a sense of users’ current understanding of AI, helping inform your communication around how AI is working. For example, if you discover that your users have a very limited understanding of AI and how it works within your product, you might need to start at a more basic level in how you communicate with them. In turn, this can inform how you approach help text, in-experience prompts, and FAQ pages.
Research around bias and fairness
We know AI systems can cause unintended harm to specific underrepresented user groups, leading to disproportionate harms and bias. This is a place where inclusive research and dedicated research with minority users is of vital importance. For example, understanding if AI-generated captions could potentially out or misgender a transgender user and how that harm could be prevented. Or, developing a deeper understanding of facial recognition technologies to better identify users with darker skin tones. When you're thinking about fairness and bias, you must talk to the population that might be affected and get their input on how to avoid and address potential harms and biases.
…
As AI continues to revolutionize products and services, UX research plays a vital role in ensuring the successful integration of AI into products and services. The capabilities of generative AI are expanding rapidly. It's critical to remember that the focus should always be on meeting user needs and providing a safe and secure user experience.
Check out our updated article on Responsible AI: Building AI Products Responsibly: Why Product Research Matters
Max Symuleski
Max Symuleski is the AI Product Manager and a former Senior UX Researcher at AnswerLab with 10 years of research experience in emerging tech and its social and cultural impacts. Max has led several projects on Responsible AI, engaging with experts in artificial intelligence and machine learning from academia, industry, and public policy to help clients better understand how they might prevent harm and mitigate potential risks. Max has also conducted in-depth research on AI chat experiences, understanding what makes topic-based conversations with experts engaging, helpful, and trustworthy to better fine-tune AI chat models. They hold a Ph.D. in Computational Media, Arts, and Cultures from Duke University and an M.A. in Historical Studies from the New School for Social Research.related insights
Get the insights newsletter
Unlock business growth with insights from our monthly newsletter. Join an exclusive community of UX, CX, and Product leaders who leverage actionable resources to create impactful brand experiences.