Financial services brands are expanding into new territories and taking a more holistic approach towards their research....
Three Common Benchmarking Metrics to Ditch and What to Use Instead
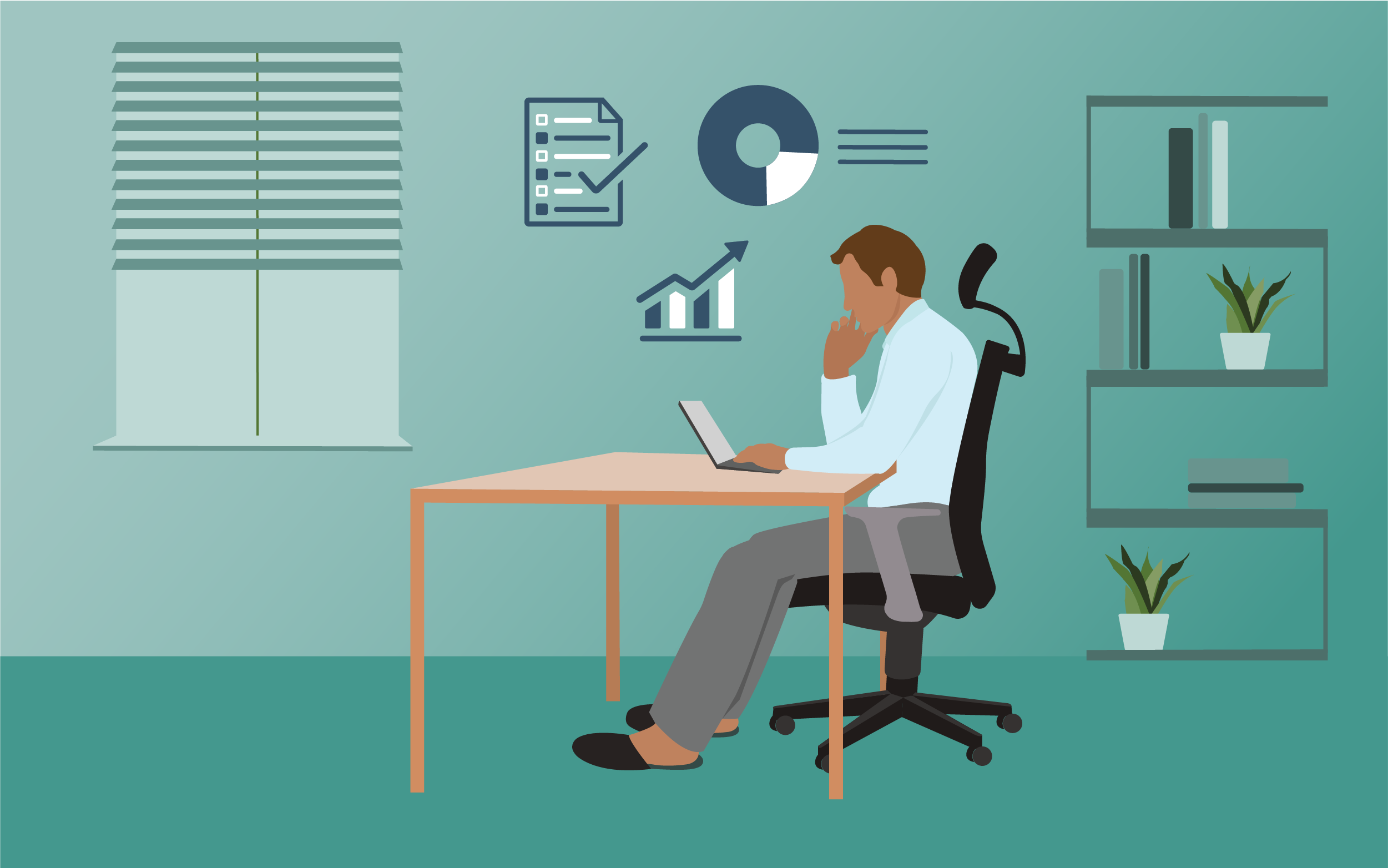
Posted by Emily Singer on Oct 16, 2020
Benchmarking research takes many forms, but at its core it’s a process of test, change, re-test. At AnswerLab, we conduct benchmarking in a variety of ways, but find that they often fall into three big buckets ranging from comparing experiences to one another qualitatively all the way to gathering data and metrics for a pure quantitative diagnostic of your product. Recently our team wrote about these three benchmarking approaches in-depth, exploring when to use them and how. Today, we’re talking about the benchmarking metrics you should (and often shouldn’t) be using to get impactful and effective insights.
There are a lot of metrics you can collect during benchmarking research; some are more common or well-known than others. Too often, teams assume certain metrics correlate strongly to product comprehension or usability, when in fact this relationship is not always direct. With moderated benchmarking studies, we recommend you ditch some of these common metrics that might not be best serving your needs. Instead, try something else for more impactful insights that will help you build better products in the long run. So what are those metrics? Why shouldn’t you use them? And, what should you be using as an alternative?
Are these benchmarking metrics still meeting your needs?
In the majority of cases, we recommend removing the following three metrics from your benchmarking plans. Not only do they present some challenges with moderated research that can influence the results, but they also don’t give you as clear an understanding of usability as you might think. Of course, every study and product is different, but we recommend taking a moment to re-evaluate what you’re collecting and make sure your existing metrics are still helping you achieve your goals.
Time on Task (ToT)
Time on task is the duration of time from when a participant starts attempting a task until a designated completion point or until they simply give up. The researcher logs a start and end time as a participant attempts each task, and then probes to understand what factors or elements contributed to how long a participant took during the process. Time on task is a standard in benchmarking research, but we think there are better ways to get at usability findings.
Time on Task can be a meaningful metric in situations where a decreased amount of time spent on a task is a clearly defined indicator of usability. For instance, say you are designing an app for new parents. You know from a prior needs analysis that your potential users want to spend as little time as possible in the app. You’re conducting a benchmarking analysis with tasks like ‘Find the nearest store that carries your preferred brand of baby formula.’ In this example, you already know your customers are motivated to spend as little time as possible, so ToT is a good indicator of your product goals and can be used effectively.
However, while it may seem like all tasks pertaining to findability would benefit from lower ToT, in reality additional time spent on some tasks can be harmless or even beneficial. For instance, if you’re refining the check-out process in your ecommerce product’s experience, a more meandering path could mean a user is finding it helpful or interesting to explore other products or features on their way to checking out. Time is only one facet of an experience, and spending more time on a task can be due to excitement or curiosity, not necessarily poor usability.
Logistically, it's not always feasible to collect ToT depending on your approach. During moderated research, if participants engage with the moderator to ask questions or think aloud about the tasks (even when asked not to), ToT is no longer valid as it doesn’t mimic how they would attempt the task in their natural environment.
If you choose to log ToT in your research, just be aware of its limitations and be clear about your objectives. Don’t simply use it as a default metric, but instead as an intentional decision based on your study goals. Collecting ToT can often lead to a false sense of certainty for benchmarking metrics, when in reality, your efforts might be better spent elsewhere to get richer, more meaningful insights.
Click Count
There are a number of opinions about the number of clicks it should take to successfully complete a task or find information on a website. For example, the three-click rule argues that most people give up after three clicks, but this is mostly unsubstantiated. While it can be beneficial to reduce click counts for some tasks, a reduction in the number of clicks doesn’t always mean users will have a better experience. More clicks doesn't always imply confusion or difficulty completing a task. Therefore, we almost always discourage using click count in benchmarking studies, because we find it’s just not a meaningful metric for usability.
Meaningful clicks are far more important to measure than just a total click count. The number of clicks doesn’t actually tell you anything concrete that will help you make meaningful changes. It doesn’t tell you what people clicked on or the order in which they clicked. For example, if someone is registering for an account and they are faced with too many registration questions and by default, more clicks, they might be more likely to abandon the process. However, the more important question is why they are abandoning the process. Are the questions irrelevant or too invasive? Understanding the motivations behind a user’s pathway and journey through a flow will give you more actionable insights than simply a click count alone.
Error Count
Error count tracks the number of specific task errors throughout a session and subsequently rolls them up into a total error count. With moderated research, we often find it’s better to take a more qualitative approach. From a moderation perspective, preparing to collect this data requires a good deal of upfront work. You have to build a list of anticipated errors, which often overlooks unknown errors that will undoubtedly be surfaced during research sessions. Coding for these errors during sessions demands a high level of focus from the moderator, which potentially compromises the quality of data, time available to probe, or note-taking capabilities.
We find it’s much more valuable to have a discussion on high-frequency errors, listing the most common errors participants make in order of frequency and some qualitative findings about the reason they’re happening. Knowing which errors are most common and understanding why they’re happening rather than just a number of errors equips you with the tools you need to make any necessary changes and subsequently, re-test for improvements.
Benchmarking metrics that help you meet your product goals
We’ve explored some common metrics we don’t think are as useful as they seem. Now let’s dive into the alternatives. We recommend swapping in or upleveling with these metrics for a richer understanding of whether or not participants are succeeding (and why), how they’re approaching tasks, and their perceptions of the experience itself.
Task Success Rate
For every task you ask a participant to complete, the task success rate reflects whether or not they successfully completed it and if they completed it with difficulty or ease. Success rate is a clear measure of usability for tasks and flows during your study. While still vulnerable to a low degree of subjectivity, criteria for success and failure are easy to define, and therefore, when you repeat your benchmarking study over time, it can provide a reliable assessment of improvement. In most cases, success rate is the main metric you should be looking at in a benchmarking study.
We typically score each task with one of the three following ratings: Success with Ease, Success with Difficulty, and Failure. As you’re preparing for sessions, make sure you have clearly defined parameters for what each of these ratings means for each task to ensure consistency and ease when scoring. Once you’re finished, you can use this data to easily calculate an overall score for each task.
Another component you may want to measure is “perceived success.” Some participants will think they completed the task successfully when in reality they weren’t able to. The difference in the number of people who believed they were successful but weren’t vs. the number of people who were actually successful can be very telling.
Description of Path
Capturing the path a participant takes to complete a task can help you identify the common approaches to the task, as well as creative workarounds and unexpected journeys.
During sessions, monitor and take note of the paths your participants take while attempting the tasks. You might consider defining the ideal path before sessions and then monitor which participants deviated from that ideal path and whether or not they were still successful. At the end of sessions, you’ll have a list of the most common paths taken, ways participants deviated from your ideal path, and qualitative findings to lend insight into the user experience when utilizing those paths. As a bonus, you’ll also have a good record of participants’ individual experiences, making it easier to review specific session recordings or pull highlights to share with your stakeholders.
Post-Task, Participant-Reported Ratings
One of the simplest but most effective metrics to gather during benchmarking is the one that comes directly from the participants’ own mouth: a self-assessed rating selected from a Likert scale.
At AnswerLab, we don’t necessarily prescribe one set of ratings for every study, but rather work with specific project teams to understand what metrics are most valuable to capture. We often incorporate rating questions around ease of use and customer satisfaction for participants to answer. Aligning these questions with your business and product goals is critical to getting valuable insights. Additionally, we almost always recommend asking a question to gauge participants’ familiarity with the task prior to the session, which can serve as a helpful piece of context in the face of high failure rates.
Every product is different, and your study might need a slightly different approach or a unique set of metrics depending on your business goals. Regardless of what metrics you’re using, benchmarking is a great way to track your product’s performance and build a better understanding of your user experience over time.
Learn more about how to approach benchmarking research with strategies and tips from our team. Need help running your benchmarking research? Contact us!
Emily Singer
Emily Singer, a member of our AnswerLab Alumni, was a UX Research Manager during her time at AnswerLab where she managed research to answer our clients’ strategic business questions and create experiences people love. Emily may not work with us any longer, but we'll always consider her an AnswerLabber at heart!related insights
stay connected with AnswerLab
Keep up with the latest in UX research. Our monthly newsletter offers useful UX insights and tips, relevant research, and news from our team.